Improving medical prediction of pneumonia cases using Deep Learning
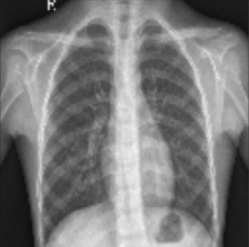
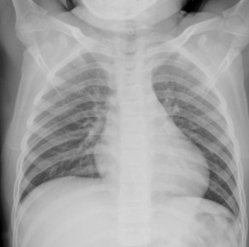
Fig1. Example of dataset image with pneumonia AND example of normal dataset. Lung X-ray with pneumonia shows opaque spots on the image.
The dataset released in 2018 by Kermany et al. has a sample of 5840 images belonging to lung radiographs labelled as pneumonia diagnosis and non-pneumonia X-ray radiographs. These images have been selected under human quality control and labelled according to an expert’s diagnosis before being validated for training in the system.
1- Dataset Preparation
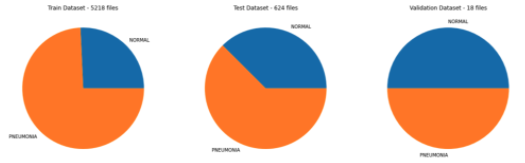
Fig 2. The dataset is subdivided into Train, Test and Validation sets.
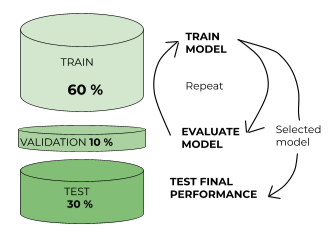
Fig 3. Division of the dataset into training, test and validation sets with the processes used for each dataset.
2- Model Architecture
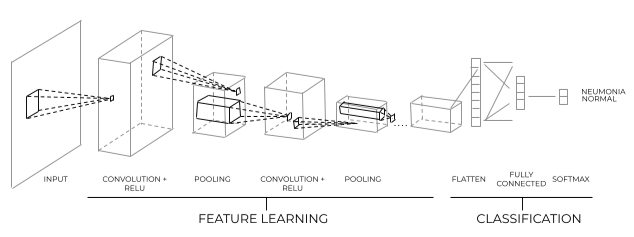
Fig 4. Schematic of convolutional neural network architecture. The convolutional layers help with feature learning, the final perceptron layers classify images with Pneumonia.
3- Evaluating the performance of the model
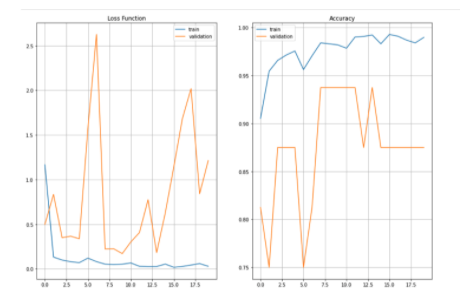
Fig 5. Loss and accuracy functions evaluating training and validation datasets.
Conclusion
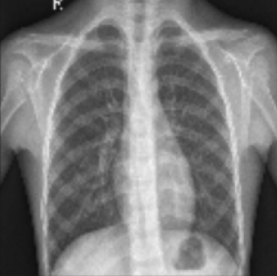
Do you want a data-driven solution?
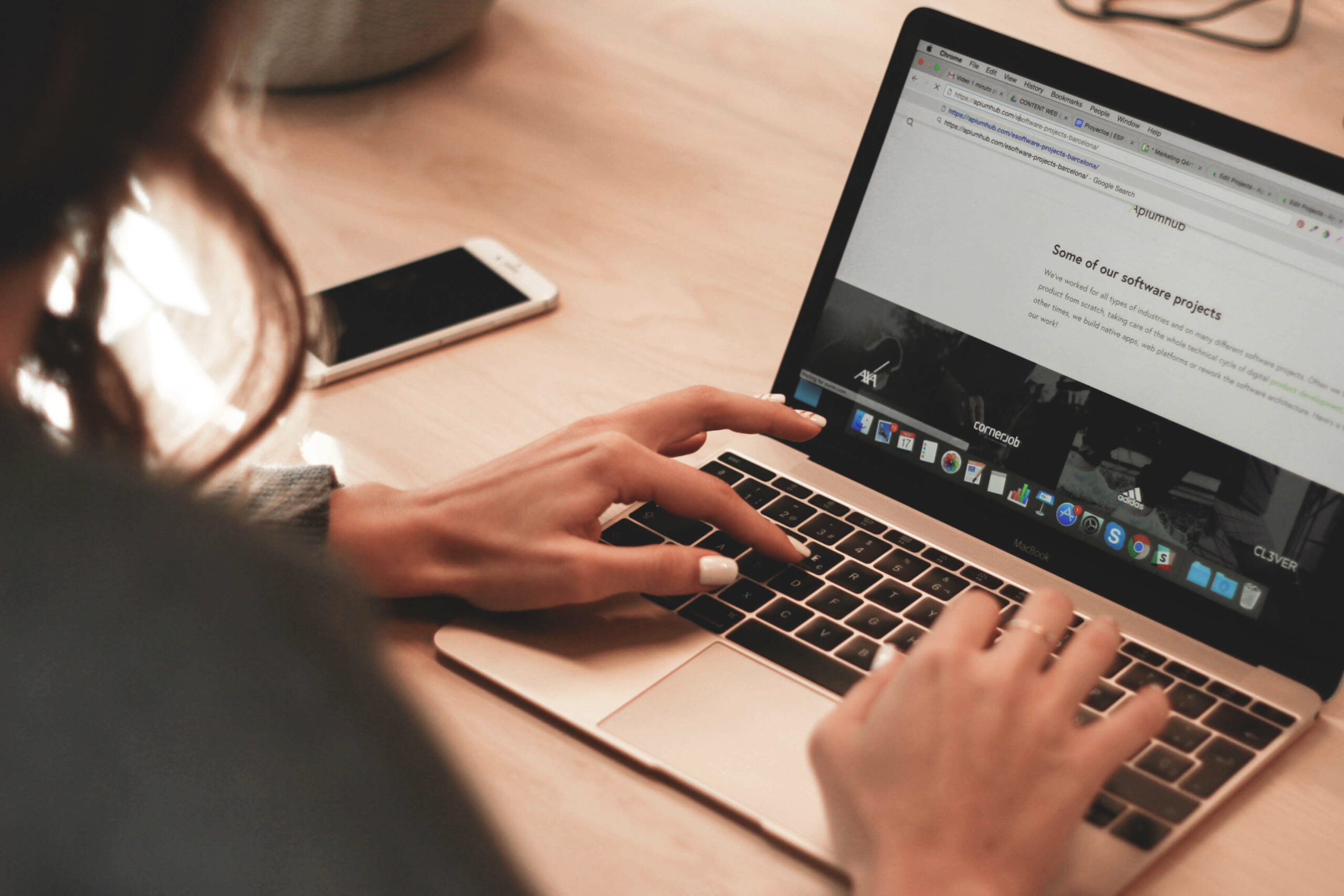