Experts in Software Architecture
Achieving software scalability, performance and cost reduction
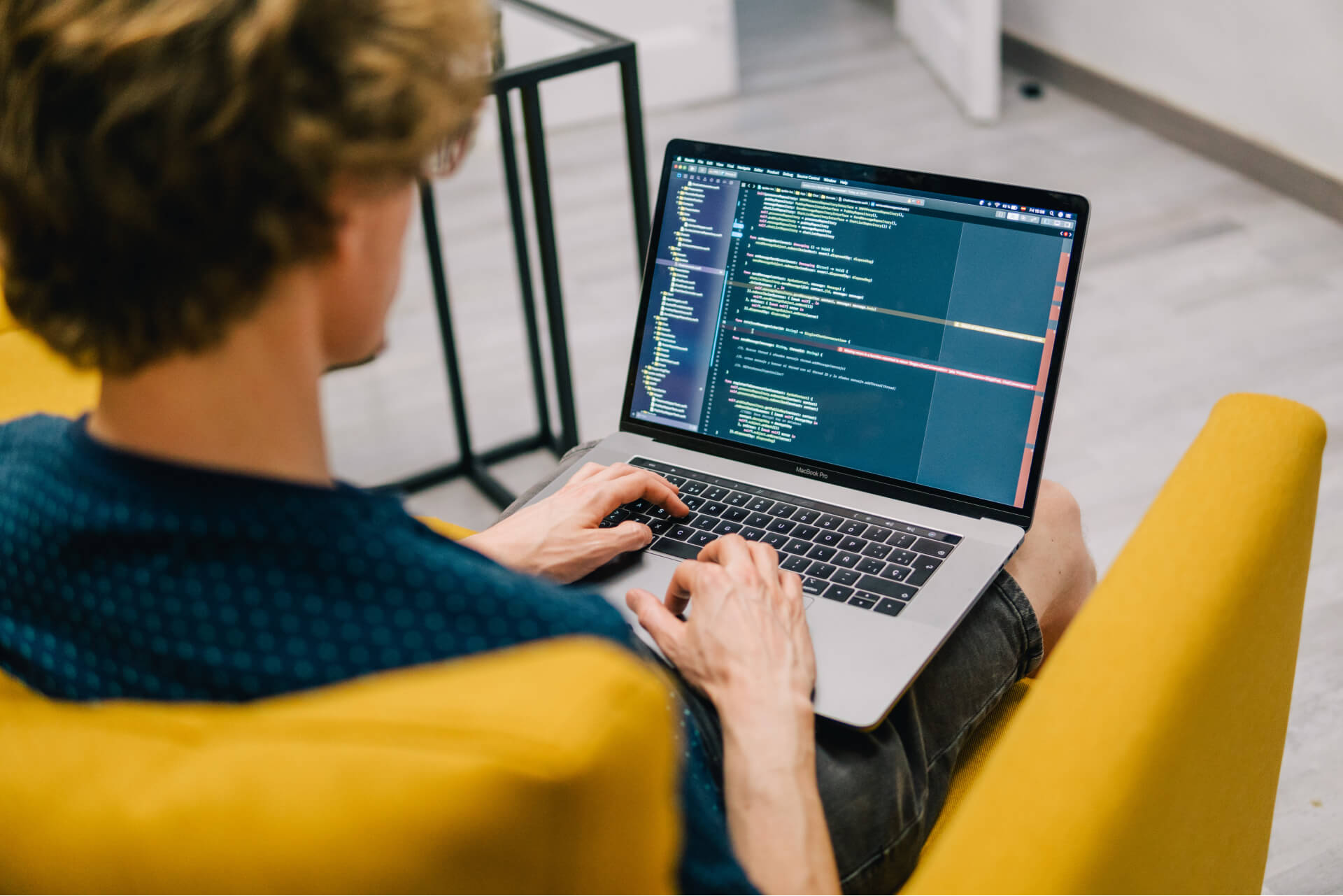
Apiumhub is a software partner that helps technical teams to deliver more robust, scalable and better performing working software on time by improving their estimation, development, QA, automation and delivery practices.
We are experts in Hexagonal Architecture, Event Driven Architecture, Microservices & DDD
We possess the expertise to enhance your team's efficiency and instill a sense of pride in both their accomplishments and how they achieve them.
01
Software Teams Mentorship
02
Software Delivery Quality
03
Delivery Time Reduction
A Dedicated Development Team for your project needs
We build custom software development
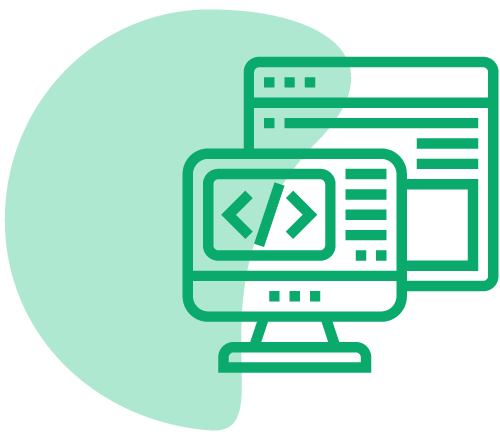
Web Development Services
We build robust web platforms, having expertise in a big range of technologies, such as: Scala, Java, Kotlin, Nodejs, .Net, React, etc. We help your project either take off or evolve.
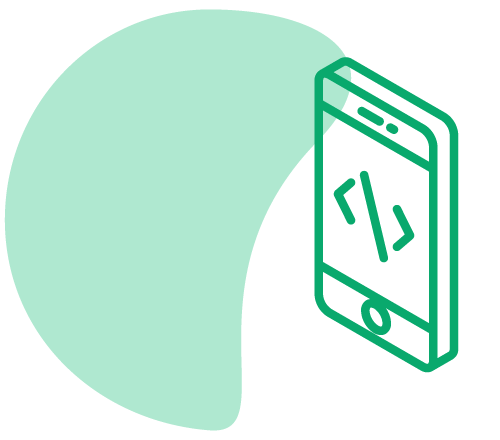
Mobile App Development
We develop native iOS and Android apps with Kotlin and Swift that add value to your business. We pay special attention to software architecture, scalability and best practices.
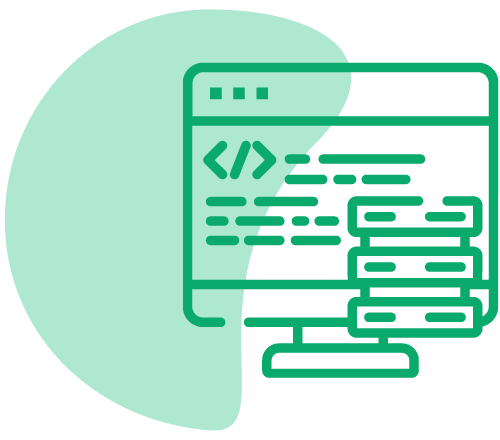
Software Architecture
We define and implement the software architecture that best fits your digital product to ensure platform scalability, high performance and cost reduction. We build custom software.
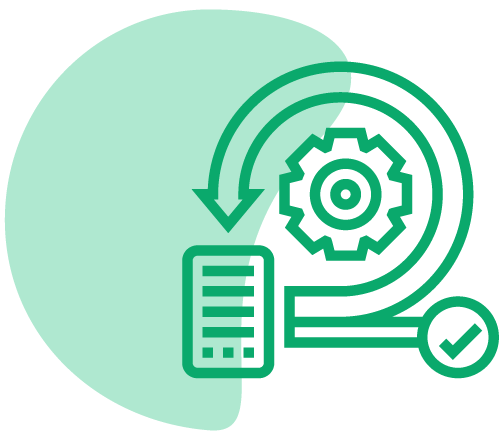
Agile Product Owner
We accelerate innovation and ROI. We have enormous experience in defining, building, and delivering high quality digital products. Transparency and communication are key to ensure project success.
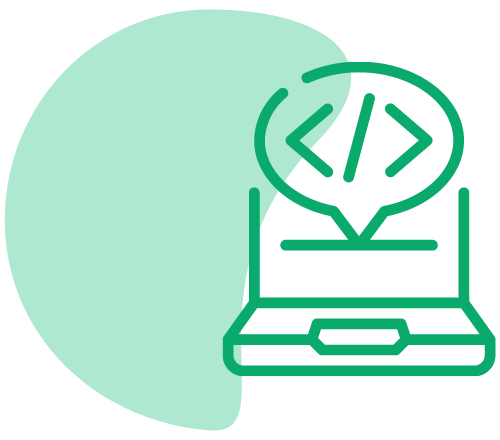
DevOps
We have enormous experience in DevOps consultancy, environment building and automation, dockerization, deployment automation, continuous delivery and performance tests.
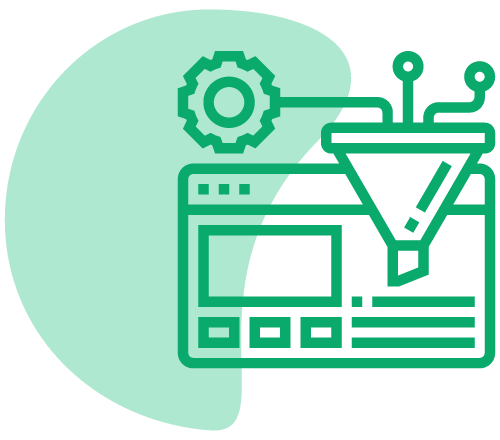
QA Software Automation
We know that quality is more than testing: our QA consultants proactively identify key problems, design processes and deliver extensive reports with actionable insights and recommendations.
Testimonials
what our clients have to say
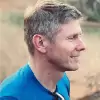
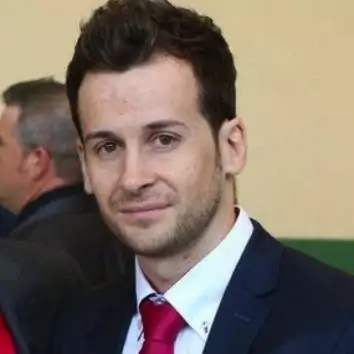
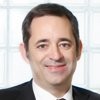
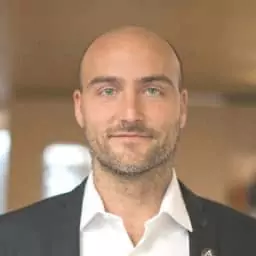
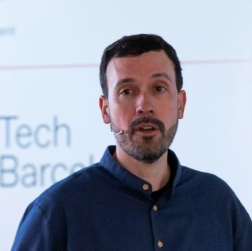
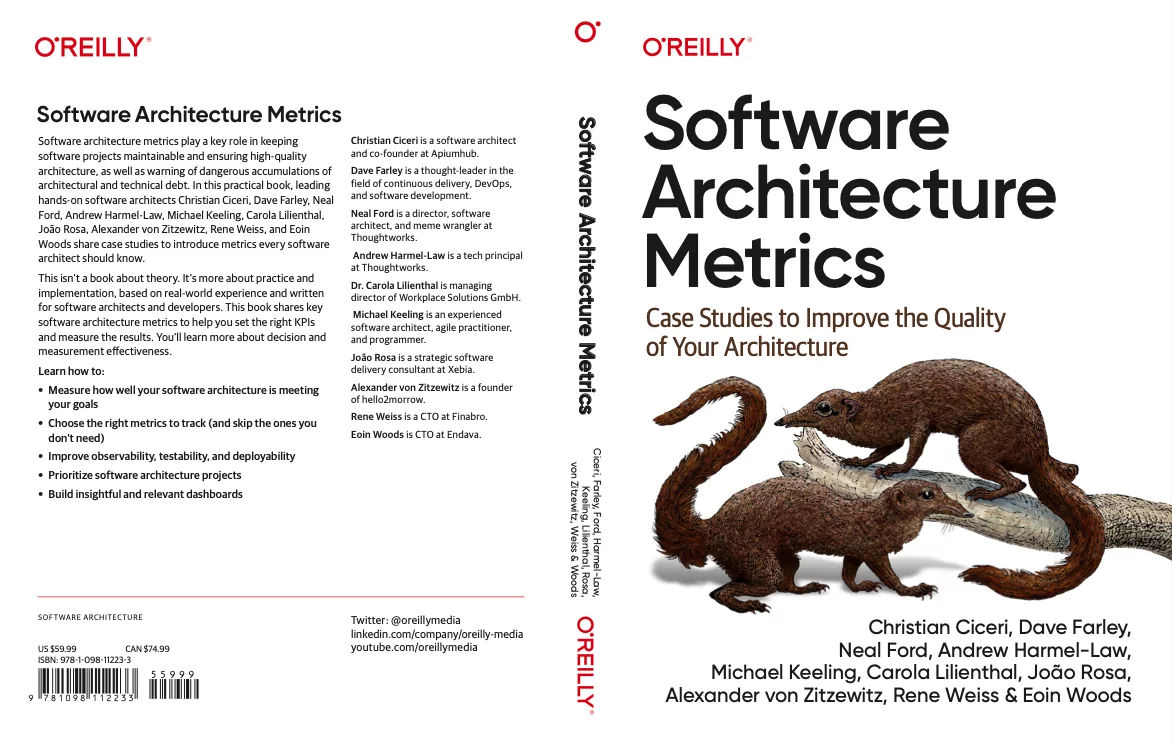
Get Our Book:
Software Architecture Metrics
Software architecture metrics are key to the maintainability and architectural quality of a software project and they can warn you about dangerous accumulations of architectural and technical debt early in the process. Written by practitioners for software architects and software developers eager to explore successful case studies, this guide will help you learn more about decision and measurement effectiveness.
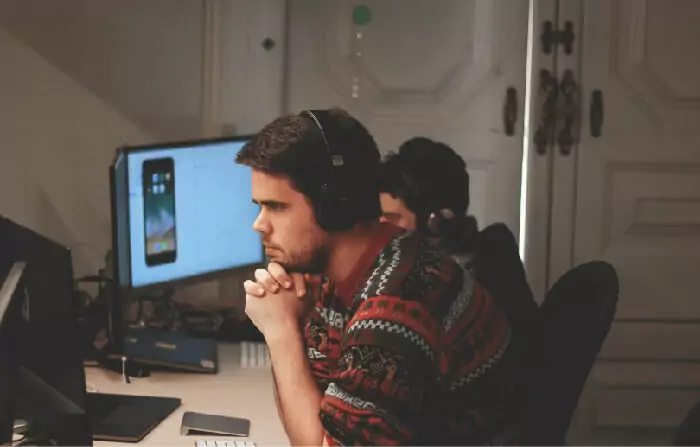